Application logging is an essential technique to both confirm normal operations and determine root cause when an incident occurs. With modern applications becoming increasingly more complex the volume of log data produced has increased exponentially. This presents a challenge to businesses, logging data is undoubtedly useful but it is also expensive to process and store. This is true whether the log processing and aggregation is managed in house or handled by one of the many SaaS providers. The greater the volume of logs, the bigger the cost.
Not All Log Data Is Important All The Time
When everything is ticking along smoothly, logging is used to confirm that transactions are being processed in a prompt and error free manner. The actual content of the log message is not so important, instead the number of log messages per time period is often used as a metric; for example web server access logs. However, when an incident occurs every log line is potentially very important and under these circumstances there is no such thing as too much logging. This is a difficult balancing act to achieve, having enough information in logging to diagnose problems but not having too much log data so that the cost is prohibitive. With some applications it is possible to dynamically change the logging level, switching to more detailed logging when an incident is detected but this is a bit like closing the stable door after the horse has bolted; the important piece of information you need was not captured in the logs when the incident was triggered.
Two-Tier Storage
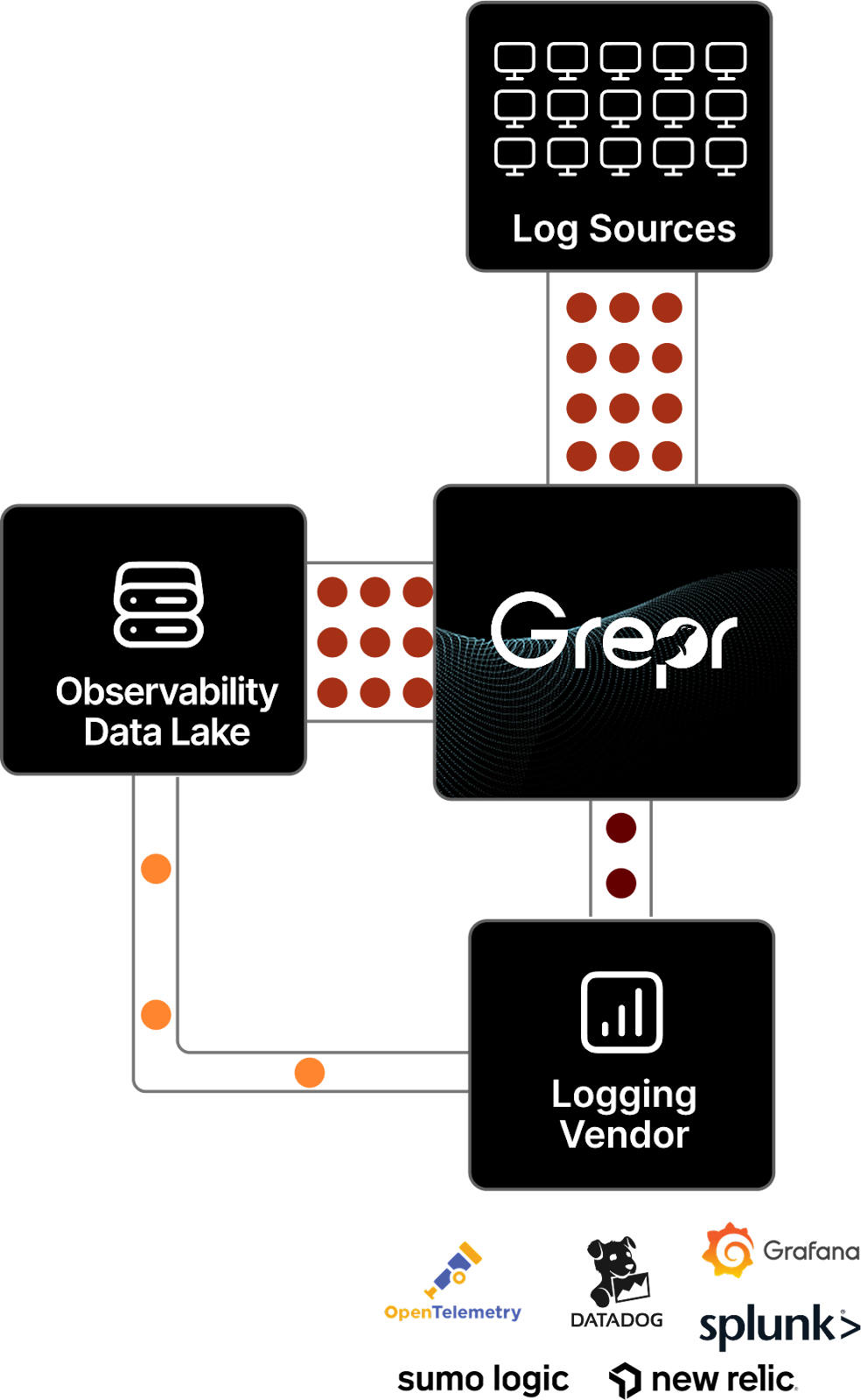
Grepr slips in like a shim between the log shippers and the backend storage and aggregation server. It uses machine learning and a rules engine to determine what to forward to the backend and what to keep in low cost storage.
The machine learning algorithm automatically detects similarity patterns between the log messages then instead of passing every message through it periodically sends a summary of similar messages. Along with the summary of similar messages Grepr also sends samples of every pattern, therefore any unique or very infrequent message patterns are passed through without aggregation. This ensures that no important detail is not immediately available in your chosen log tooling.
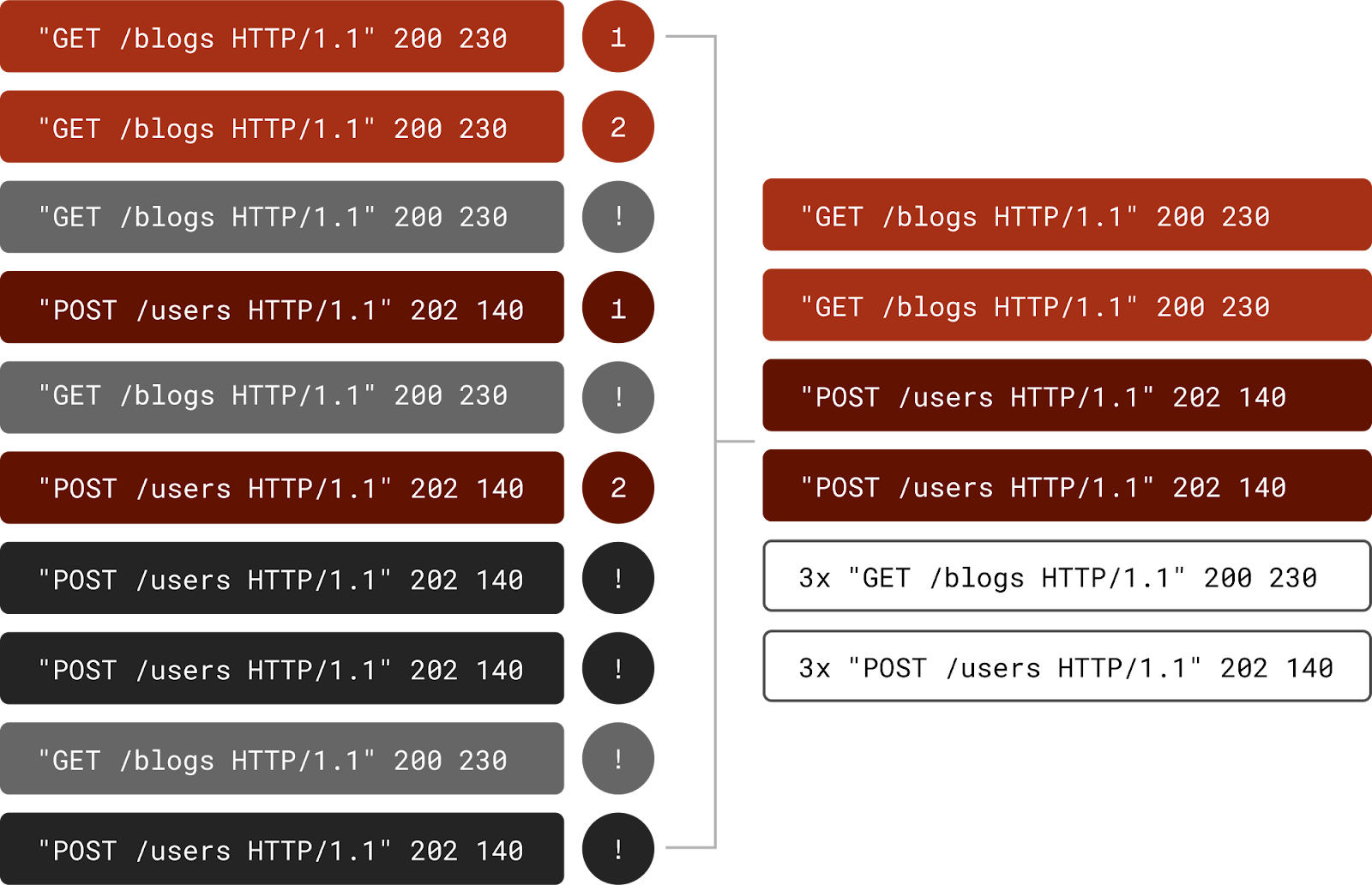
The rules engine allows for fine tuning of what stays in low cost storage and what passes through. This typically results in a 90% reduction in log volume sent to the backend which realises a significant saving on log processing costs.
Nothing is discarded, every message either gets passed straight through when it matches a rule or it gets summarised and retained to low cost storage.
Dynamic Detail
The messages retained in low cost storage are not locked away, they are always available when needed. Grepr provides both a web interface and an API to query these logs. However, engineers do not want to look in two places when troubleshooting an incident; they want to stay with the log tool they know and love. This is where the magic of Grepr’s dynamic backfill comes to the rescue. The log messages relevant to the incident are quickly backfilled into the log aggregation server; dynamic low level detail on demand at low cost.
Start Saving Now
Give Grepr a spin and see how easy it is to start saving 90% on your logging services cost with zero interruption to your existing workflows. Stop worrying about achieving that fine balance between logging visibility and cost. You can now log in more detail without worrying about blowing the budget. Feel secure that you have all the log detail you need to deal with any incident in the tool you are already familiar with using Grepr dynamic backfill on demand.
More blog posts
All blog posts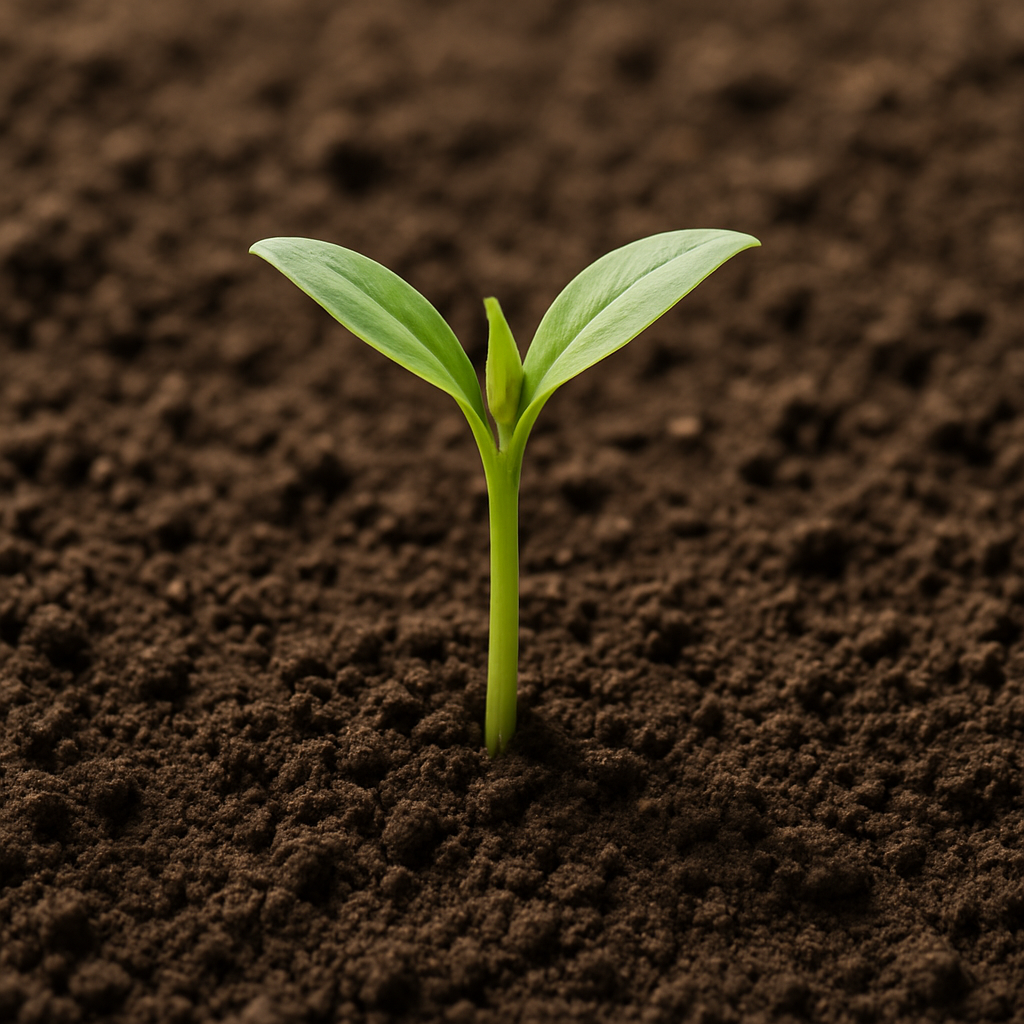
Dirt-cheap, infinite, queryable storage
6 ways Grepr Optimizes the Logs Data Lake
