The log retention dilemma
Modern organizations face a dilemma when it comes to log management. Regulatory compliance often requires retaining logs for years. For instance, the Sarbanes-Oxley Act (SOX) mandates financial institutions retain relevant records, including logs, for a minimum of seven years. Yet traditional log aggregation vendors like Splunk, Datadog, and Elastic charge premium prices for long-term log retention. As a result, many companies find themselves making impossible tradeoffs:
- Keep everything and watch costs spiral out of control
- Delete valuable data and risk compliance violations
- Implement complex, custom archiving solutions that are difficult to query
What if there was a better way? A solution that provides unlimited log retention at a fraction of the cost while keeping all your data easily queryable when you need to find it.
Enter the log data lake
A log data lake leverages cloud object storage (like AWS S3) to store your logs at pennies per GB while maintaining the ability to query when needed. The approach works by separating storage from compute - only paying for computation when you actually need to analyze the data.
What makes this approach particularly powerful for log management is the use of Apache Iceberg, an open table format that adds structure and efficiency to your log data lake. Iceberg identifies which specific files to read for a query at query planning time, making it possible to limit the scope of a query to only the files that need to be read.
The true game-changer, however, is tiered storage. Cloud providers like AWS offer multiple storage classes with decreasing costs for data you access less frequently. By automatically moving older logs to cheaper storage tiers, you can reduce costs by up to 93% compared to traditional vendors, all while keeping data queryable by Grepr when needed.
How Grepr builds your log data lake
Grepr provides a turnkey solution for building and managing your log data lake without disrupting your existing observability, security or compliance workflows. Here's how it works:
- Simple collection: Point your existing log agents to Grepr, either by dual-shipping logs from the agents to both Grepr and your existing log vendor, or by having Grepr forward the logs to your vendor.
- Automatic organization: Logs are stored in Apache Iceberg tables, optimized for efficient querying.
- Familiar querying: Use the query language of your existing vendor (Datadog queries currently supported, Splunk SPL planned May 2025) to search all your logs in your data lake, regardless of age.
The beauty of this approach is that it is all additive. You gain immediate long-term access to log data at a fraction of the cost without impacting any of your existing workflows.
Setting up tiered storage for your log data lake
One of the most powerful cost-saving features of a log data lake is tiered storage - automatically moving older logs to progressively cheaper storage classes. Here's a step-by-step guide to setting this up with Grepr and AWS S3:
Step 1: Identify the path to your data
You’ll want to identify the prefix path to your log data, sitting in your bucket. Grepr stores multiple Iceberg tables of data in S3 for each set of data. You can browse through your S3 bucket in the AWS console to find the prefix that contains the paths to the files that you care about. Keep track of this prefix, as you’ll use it in the next step.
Step 2: Create a bucket lifecycle configuration file
Create a file named lifecycle-config.json with the following content, replacing <your prefix> with the prefix you got in step 1:
{
"Rules": [
{
"ID": "Move to Infrequent Access after 30 days",
"Status": "Enabled",
"Filter": {
"Prefix": "<your prefix>"
},
"Transitions": [
{
"Days": 30,
"StorageClass": "STANDARD_IA"
}
]
},
{
"ID": "Move to Glacier Instant Retrieval after 90 days",
"Status": "Enabled",
"Filter": {
"Prefix": "<your prefix>"
},
"Transitions": [
{
"Days": 90,
"StorageClass": "GLACIER_IR"
}
]
}
]
}
This configuration defines two tiers with progressively lower costs:
- Standard S3 for recent logs (last 30 days)
- S3 Infrequent Access for medium-term logs (30-90 days)
- S3 Glacier Instant Retrieval for older logs (beyond 90 days)
Step 2: Apply the configuration using AWS CLI
aws s3api put-bucket-lifecycle-configuration \
--bucket <your bucket here> \
--lifecycle-configuration file://lifecycle-config.json
That's it! Grepr will now automatically store your logs in Apache Iceberg tables within your S3 bucket, and AWS will handle moving the data between storage tiers based on age.
Cost comparison: Traditional vendors vs. Log data lake
As an example of Grepr’s ability to query on low cost storage, let’s examine the differences in costs for an example workload. Say you’re storing 1TB of logs per day:
Traditional logging vendor (in this case, Datadog Flex Logs):
- 1TB per day = approximately 1 billion log messages per day (assuming 1KB per message)
- 1 billion messages × 365 days = 365 billion messages stored
- 365 billion messages × $0.05 per million messages per month × 12 months = $219,000/year (Based on Datadog's Flex Logs pricing at $0.05 per million messages stored per month)
Grepr tiered log data lake approach:
- Assuming 50% conservative compression log using Parquet format.
- S3 Standard (30 days): 30TB × 50% compression × $0.023/GB × 12 months = $4,140/year (AWS S3 Standard pricing: $0.023/GB/month)
- S3 IA (60 days): 60TB × 50% compression × $0.0125/GB × 12 months = $4,500/year (AWS S3 Infrequent Access pricing: $0.0125/GB/month)
- S3 Glacier Instant Retrieval (275 days): 275TB × 50% compression × $0.004/GB × 12 months = $6,600/year (AWS Glacier Instant Retrieval pricing: $0.004/GB/month)
- Total: $15,240/year (93% savings)
The cost difference becomes even more dramatic as your retention period extends beyond one year. For example, keeping 5 years of data with Datadog Flex Logs would cost approximately $1,095,000, while the Grepr data lake approach would cost approximately $76,200 for 5 years of retention. Furthermore, we’re working on ways to enable the use of archival tiers, like S3 Glacier Deep Archive to further exponentially reduce the cost of storage.
Query Performance
Of course, querying data from a data lake will be slower than querying data from a full-text indexed store. However, because Grepr uses massively parallel processing in the backend to execute queries, and combined with Apache Iceberg’s and Apache Parquet’s capabilities to quickly filter down the parts of specific files that need to be scanned in query, Grepr can answer 98% of users queries in under 10 seconds. The hard work is in making sure the data is partitioned and laid out efficiently in the data lake to enable this kind of performance, combined with Grepr’s ability to optimize the queries that users make.
Beyond the Log Data Lake
Beyond reducing the cost of storing logs for long term retention using a data lake, Grepr also helps reduce the volume of data that goes to your vendor by using log summarization. When combined with the data lake, the full Grepr solution has been proven to reduce total logging costs by over 80% with minimal effort and impact to existing workflows.
Getting started with Grepr
Setting up dirt-cheap, infinite log retention with Grepr takes just minutes:
- Sign up for a Grepr account at grepr.ai
- Configure your log agents to send data to Grepr
- Set up your S3 bucket with tiered storage as shown above
- Continue using your existing tools with the knowledge that all your logs are safely stored and queryable
Don't let expensive log retention force you to delete valuable data. With Grepr and a properly configured log data lake, you can have it all: unlimited retention, full queryability, and costs reduced by up to 93%.
Ready to transform your log retention strategy? Visit grepr.ai to learn more or sign up for a free trial today.
More blog posts
All blog posts
Aggregate my log volume by 90%, yet still find anything I need? How is that possible?
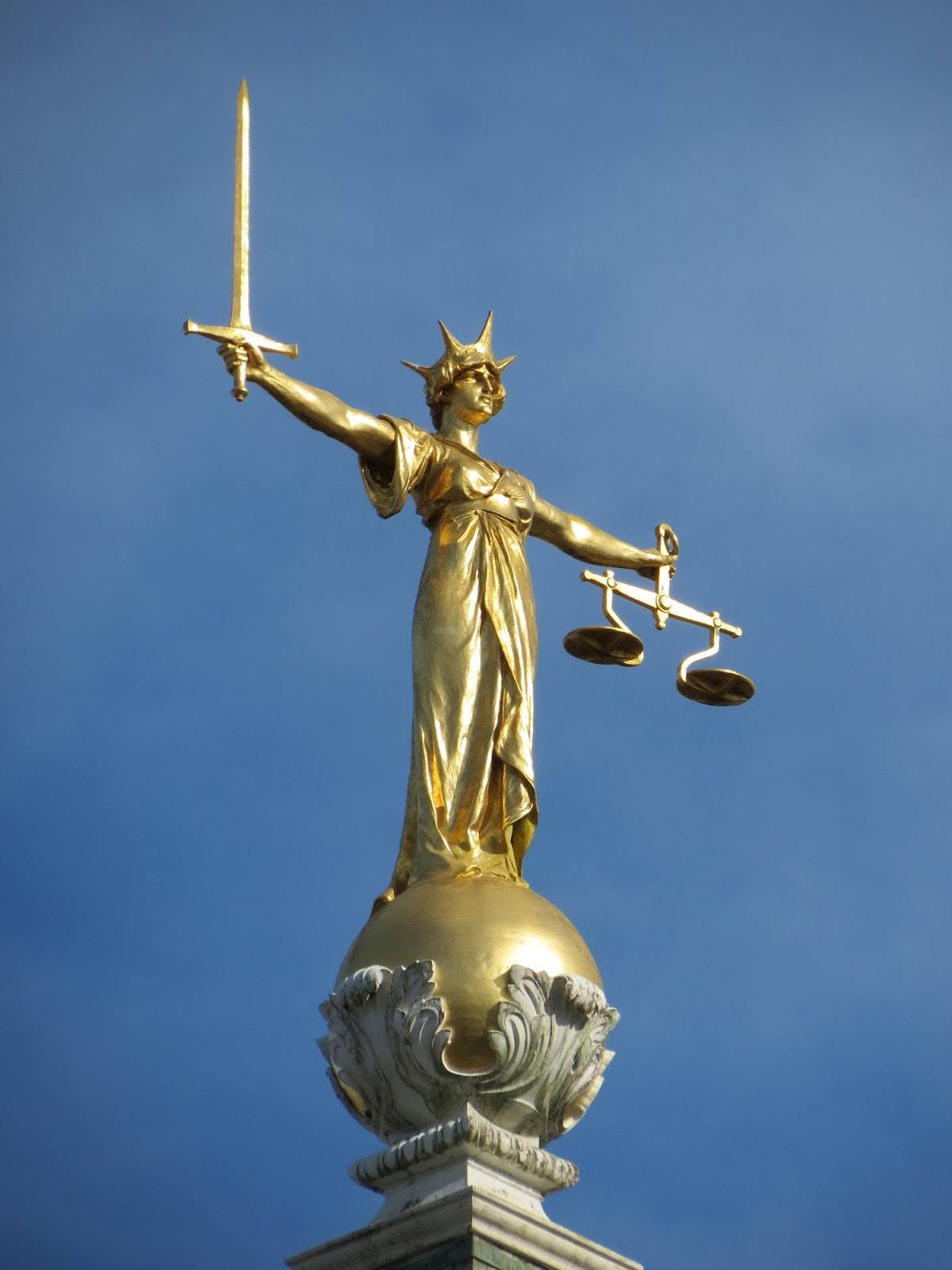
All Observability Data Is Equal But Some Is More Equal Than Others
.png)